Insufficient data may not compute, but it still loves you.
I train neural networks to imitate real-life human things, from fortune cookies to to Harry Potter fan fiction to guinea pig names. Unlike traditional computer programming where a human programmer makes up rules that the program has to follow, when I train a neural network, I only have to give it the dataset - and the neural network makes its own rules.
The neural network always tries its best, but sometimes it has trouble figuring out what’s going on. One frequent problem: insufficient data.
When I train a neural network, it needs to see lots of examples before it can form a general rule about it. Otherwise, the best it can do is to memorize each individual example. This is why neural network researchers like really big datasets - and many of the neural network’s most realistic results (craft beers, metal bands, names of stories) have happened when I had tens or hundreds of thousands of examples in my dataset.
Here’s what happens when there is not nearly enough data.
A while ago, I trained a neural network to generate pick-up lines. The results ended up being oddly charming in a weird sort of way, since there wasn’t enough data for the neural network to pick up on the terrible puns and wordplay. Prof. Amita Kapoor of the University of Delhi contacted me, saying she would collect some better-quality romantic lines for me - and she sent me 100 of the most flowery romance lines you could imagine, lines from Shakespeare to classic Indian epics, lines like “Shall I compare thee to a summer’s day?” and “Love, like the magic of wild melodies, Let your soul answer mine across the seas.”
They were flowery, but there weren’t nearly enough of them, and when I trained a big-brained neural network (512 neurons per layer) to generate them, it was so smart that it quickly learned to memorize the lines and spit them back (slightly garbled) at me.
Love, oh, love is flome is night, only light. And if my love could grow wings, I’d be soaring in fain
My fading face lights up when you look at me, and my physicians think this ailitilu hour or two - is gone.
Shall I compare thee to a summer’s day? Thou art more lovely and pleasant than a bright summer day
I am not interested in being a star. I just want to be wond.
So, I tried to handicap the neural network by turning off some of its neurons during training. It’s a technique called “dropout”, and the idea is that to memorize a long phrase, a neural network has to have a bunch of neurons working together in a Rube Goldberg-style mechanism. Knock a bunch of neurons out at random, though, and the neural network has to resort to simpler, more general rules that only depend on a few neurons at a time. No more memorization, in theory.
A dropout of 0.5 (50% of the neurons knocked out at random) slowed the neural network down a bit, but didn’t stop it from memorizing entire phrases.
And love will last as pure and whole As when he love is life and mere, And put your harsher moods and starry skies.
What the hell is with me always, wherever I go.
A hoppy farther with the dawning soul.
TAKE, O take those lips away week you roaming? O, danker is wonderful, but you can’t curl up with it on a cold night.
So I decided to be harsher. I tried a dropout of 0.8 instead. At any given time during the training, 80% of the network’s neurons are completely nonfunctional, and it doesn’t know in advance which 80%. Furthermore, I restricted its memory to only 15 characters at a time (a couple of words, no more).
That did the trick. The neural network had a much harder time.
Lehed wres deer than ghon the deer the seing bite; souit the weent witite: I fot and done I ghimh thos the seosd.
I af thot sra hoire and that ghot i romer to kron o faith the seyees co ler me In hoald gotlelt, as nise, A ou morer in beeralom is mooud worl the cowe, wo mace tho haw roy note, I your che pin’ goisid, it roriru, bu cimh fous otey thet thas dosl oc my love an yiut, pawk nive.
Ats the worg, wu rive dy cotesilg, And you one I co lhe I on tire lovel the love re nhen.
I let it try for longer.
The that sondl with her that the osle thar srash, Yau srave, the your is ond the krith me whend is thouth the thon wotk fha lenl, of love, The that the ass hee sraand, The sarl of love ass a dann, I conkd in me whot wyerh hes in ilshong, To buon vomer the bangs of the lragiog feay…
I tote co that the your whame wirh you love my sins u dose your i khour af the mesatif.
And longer.
Fouly laved love ass wonl and mome the basy . of pald wores to bann at mesk the whes you hound sore.
I darfer worr at is is fongitgh, worlhing of where of hire and woold and thon the klom datkire as love it thit that I sheat cath the nreeser wosd it In the bner beekrern and morrsaren, What and mering of the mire the sweat love of your donetise fart, py the ward nor the will is whotever me broye
Litht, bot is phising srich and to brang the dawrted, O hove ar came and samile, I lret.
And longer.
The wanging som shongh my wisging thote, I cowner to the nights and made the gatttir to lise it wore you your live, I hal, derans, My that slang her mefe.
I he would your love;
By love, my day, To canger worrd ase voud on love.
Finally, it was clear that I had slowed the neural network down just a bit too much. The only relevant word it had managed to consistently spell was “love”. (Well, “fart” too, but I’m beginning to expect that of a neural network)
I tried again with the same harsh dropout settings and short memory, but this time instead of giving the neural network letters to work with, I used this framework that lets it use syllables as its building blocks. It learned more words! Everything is words now!
if dreams the is is the love love and in are., the our than of shall with to love in that would bounded o with the who thee mine help nor alive the it, day, charms of is love and youth the to sweet nor have as that fair a my to that starry me have never, star were grave it, and and both, one i to the you and the are love the with re the, i my of prov of but it some on ter have from love t so joy at from your side hands some not bring about of your spir!
I’m not sure this is where we want to be, but at least it sounds happy.
Even if the neural network never did learn to be both original and coherent with this tiny dataset, all was not lost! In fact, it got to help write an actual opera.
Its first weird attempts turned out to be exactly what Klara Kofen of the Waste Paper Opera Company of Birmingham, England needed for the voice of an android, taught to speak by a lonely inventor.
The neural network’s lines such as
I dist love, Whatever fockle tongues may say.
My love it flomy pass to be human.
Might I starl with the dawning soul.
A haul, I frow it sture and in wenther do I remember my faithfulness, now I remember my faithfulness, now I remember my faithfulness, now I remember my faithfulness, now I remember my faithfulness, now I remember my faithfulness, now I remember my faithfulness, now I remember my faithfulness, now I remember my faithfulness…
and more, are now set to music in ‘i’ The Opera, the first-ever opera that features neural network programming. Read a review here, and watch the whole thing performed at the Tête à Tête opera festival here.
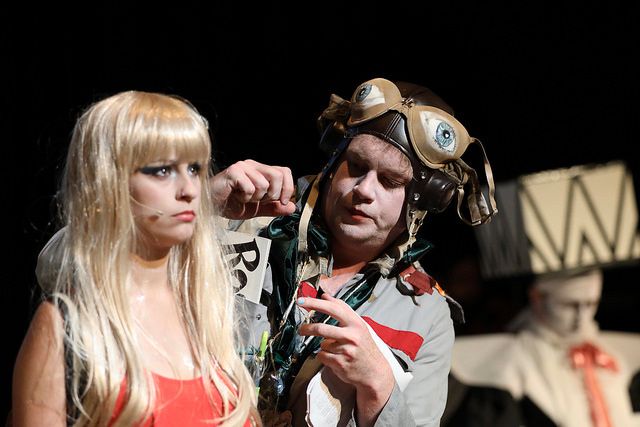
[image: The Android (Anna Palmer) and The Inventor (Benjamin Kane). Photography by Claire Shovelton]
Become an AI Weirdness supporter to get bonus content! Or become a free subscriber to get new posts in your inbox.